In today’s fast-evolving industrial landscape, the drive for efficient, data-driven decision-making has never been more crucial. Enter Causal AI, a revolutionary force reshaping how industries embrace digitization.
At its core, causal AI enables companies to understand correlations and the actual cause-and-effect relationships within their data. This deeper insight empowers businesses to make more informed decisions, optimize processes, and predict outcomes with unprecedented accuracy. Stuart Frost, CEO of Causal AI company, Geminos, explores how Causal AI will benefit industries as they compete in an increasingly data-centric world.
Understanding Causal AI
Causal AI is quickly becoming a cornerstone in the way industries approach data analysis. While traditional data analytics often focus on identifying patterns and correlations, Causal AI digs deeper, aiming to uncover the root causes behind these patterns. This understanding enables industries to make smarter decisions, pinpointing the true drivers of performance and change. But to grasp what makes Causal AI so revolutionary, it’s essential to differentiate between mere correlations and genuine causation and to explore the mechanisms that enable Causal AI to function effectively.
In the industrial sector, the distinction between causation and correlation is critical. Correlation indicates a relationship between two variables, meaning they often move together. However, correlation does not imply that one variable causes the other to change. This is where many businesses fall into traps; they make decisions based on assumed causes, only to find that they’ve addressed symptoms rather than root problems.
Causal AI helps identify these cause-and-effect relationships, going beyond the surface to drive more precise and effective industrial strategies. It’s like having a map that shows not just the roads but also which ones actually lead to your destination.
“Causal AI employs several methodologies to identify and analyze causal relationships,” says Stuart Frost. “One of the primary methods is causal inference, which uses statistical models to determine cause-and-effect links. This method goes beyond traditional statistical techniques by focusing on how variables interact in their natural settings.”
Graphical models called DAGs (Directed Acyclic Graphs) are a cornerstone of Causal AI. They represent the probabilistic relationships among variables. These models help in mapping out potential scenarios and understanding how changes in one variable affect others and they are a great communication tool for business analysts, data scientists and subject matter experts. Then there’s structural equation modeling, which combines statistical data with causal assumptions to model complex relationships. This approach allows industries to build comprehensive models that reflect real-world complexities.
Together, these methods equip industries with tools to not only identify causation but also to simulate the outcomes of various decisions, leading to optimized processes and forward-thinking strategies.
Impact of Causal AI on Industrial Processes
Causal AI is driving a paradigm shift in industrial processes, empowering businesses with actionable insights that direct their operational strategies. As organizations strive to enhance efficiency and effectiveness, Causal AI stands out with its focus on cause-and-effect, enabling industries to not just react to changes but anticipate and influence them. Let’s explore how it’s reshaping key areas like maintenance, supply chain, and quality control.
Notes Frost, “Imagine a factory floor where machines can predict when they need repairs. Causal AI makes this possible by offering insights that conventional analytics can miss.”
By understanding the causal links between machine usage and potential failures, industries can switch from reactive maintenance to predictive strategies. This ability to foresee and address issues before they escalate reduces costly downtime and boosts overall efficiency. Maintenance schedules become more dynamic, adapting to real-time conditions rather than routine checks, ensuring machinery operates at peak performance with minimal interruptions.
The supply chain is the heartbeat of manufacturing operations, yet it is often vulnerable to disruptions. Causal AI helps untangle the complexities of supply chain dynamics by pinpointing the causal factors that influence production and logistics. It sifts through vast datasets to identify hidden patterns, providing businesses with a blueprint for optimizing their supply chains.
Maintaining high-quality standards is crucial in any industry. Causal AI strengthens quality control by moving beyond superficial data patterns to reveal the underlying causes of defects. By identifying and addressing these root causes, businesses can implement improvements that prevent recurring issues. This proactive approach not only enhances product quality but also reduces wastage and recalls, leading to substantial cost savings. It is like having a digital detective on hand, ready to solve the mystery of defects before they affect the final product.
Challenges and Considerations
As industries embrace Causal AI to drive digitization, they face numerous challenges. From overcoming data obstacles to navigating organizational culture shifts, understanding and addressing these issues is crucial. This section explores these key challenges and offers insights into tackling them effectively.
“Handling data in the context of Causal AI is no small task. Data must be high-quality, unbiased, and free from noise to produce accurate outcomes,” says Frost.
Noise in data can act like static on a radio, interfering with the clear signal you’re trying to capture, which in AI terms translates to misleading insights. To combat this, industries are employing rigorous data-cleaning methods. Pre-processing data with tools that detect and filter out noise ensures that only relevant, clean data is used in modeling.
Bias in data is another formidable hurdle. Bias can skew results and lead to faulty conclusions, much like a biased umpire skewing the outcome of a game. To mitigate this, Causal AI’s graphical models can be used to identify and eliminate potential sources of bias.
Integrating Causal AI into existing frameworks requires more than just technical adjustments. It demands a cultural shift within organizations. Resistance to change is common, much like how a ship resists a change in course despite needing to head in a new direction. Overcoming this inertia requires strong leadership and a clear vision of the potential of Causal AI.
Education is a key component in driving this cultural change. By investing in training and development, organizations can build a workforce well-versed in AI technologies. When employees understand the benefits and workings of Causal AI, they are more likely to embrace it. Additionally, creating cross-functional teams encourages collaboration, fostering a shared sense of purpose and breaking down silos that might resist new technology.
Organizational structures may also need to evolve. Decision-making can no longer rely solely on intuition but should be data-driven. This shift can be likened to a transition from gut-feel navigation to compass-guided travel. Companies that adapt by fostering a culture of data-driven decision-making often find themselves more agile and competitive.
Causal AI is revolutionizing industrial digitization, offering a profound shift in how industries operate. By unveiling the cause-and-effect dynamics entrenched in vast datasets, it facilitates intelligent decision-making and strategic planning. This technology pushes past traditional analytics, allowing industries not only to react but to anticipate changes, streamlining processes across maintenance, supply chain, and quality control.
As the industrial landscape continues to evolve, the integration of Causal AI with emerging technologies like IoT and big data remains crucial. This convergence promises enhanced operational efficiencies and innovative pathways. Industries that embrace Causal AI now secure a competitive edge, paving the way for future advancements.
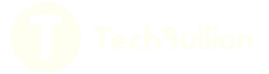
Comment here